AI and Innovation Part 2
- Liz Palisin
- Feb 4, 2023
- 11 min read
Updated: Jul 20, 2023
With AI, the sky's the limit.
At least that’s what we covered in the first part of our AI and Innovation series. In that article, we outlined the basics of AI for business and underscored AI’s potential to impact all areas of an organization.
With this entry, though, it’s time to start moving from the potential to the practical. In Part 2 of our 3-part series on AI methodologies and strategies, we cover:
The capabilities enabled by implementing AI
Straight talk on what AI still can’t do for businesses
4 common strategies for activating AI-along with real-world examples of these strategies in action
The 3 key ingredients required for AI strategic planning success
Using these resources, your organization can start down a path toward harnessing AI’s full potential. So, read on below and begin your journey from AI’s possibilities to an AI-powered reality.
What AI Can Do: New Methodologies and Capabilities
With advanced analytics, complex algorithms, machine learning, and deep learning, it’s clear that artificial intelligence is capable of revolutionizing an array of activities for both businesses and consumers.
Still, you might wonder: What does that actually look like inside an organization that’s able to leverage AI? To answer that, it helps to understand how AI’s practical solutions unlock new methodologies like those below.
Ultimately, as you’ll see though, AI doesn't’ just make possible new capabilities-it also engenders a new organizational culture to match.
AI Unlocks Collaboration and Encourages Interdisciplinary Work
One of AI’s turnkey offerings is automating business processes, including some of the most tedious or time-consuming tasks. That means talent in departments everywhere from IT and HR, to finance and marketing, get time back to focus on creative problem-solving through collaboration.
But it’s not just AI-driven output that changes how business gets done. The input involved in developing AI-based solutions encourages interdisciplinary work and drives innovation to solve for widespread inefficiencies and pain points. All told, this can translate to achieving maximum results.
As a team of McKinsey analysts write in HBR, “AI has the biggest impact when it’s developed by cross-functional teams with a mix of skills and perspectives. Having business and operational people work side by side with analytics experts will ensure that initiatives address broad organizational priorities, not just isolated business issues.” Plus, when a more diverse set of stakeholders are involved, the chances of widespread adoption increase.
AI Improves Decision-Making Across the Organization
When employees across a business can leverage AI, businesses enable improved decision-making power at every level of the organization. This shift can’t be undervalued: Employees can make decisions more quickly thanks to powerful data processing provided by AI. Plus, rather than rely on input from more senior team members, they can save time by making choices more independently.
An Example of AI-Backed Decision-Making
To understand the chain reaction this can have, consider this example, provided by McKinsey researchers.
Optimizing event scheduling is no easy task - especially when you’re trying to accommodate a large number of attendees and stakeholders, each with their own preferences and conflicts. Accounting for all these people and factors manually relies heavily on instinctive decisions, rather than actual hard data. And it’s no wonder why - there are simply too many possibilities for a human to consider all of them. Plus, even when they enlist the help of superiors, these senior members are typically also relying on intuition.
To change all this, an organization replaced manual scheduling methods with a new AI system. This system was capable of quickly analyzing millions (yes, millions!) of scheduling permutations and ranking the best possible scenarios for each participant.
These outputs equipped planners with information they could act on, independently and without the need for input from leadership. Instead, interactions between employees could focus more heavily on creating the best possible experiences at these events - not just when to hold them in the first place.
Motivate Agile, Flexible, and Innovative Thinking
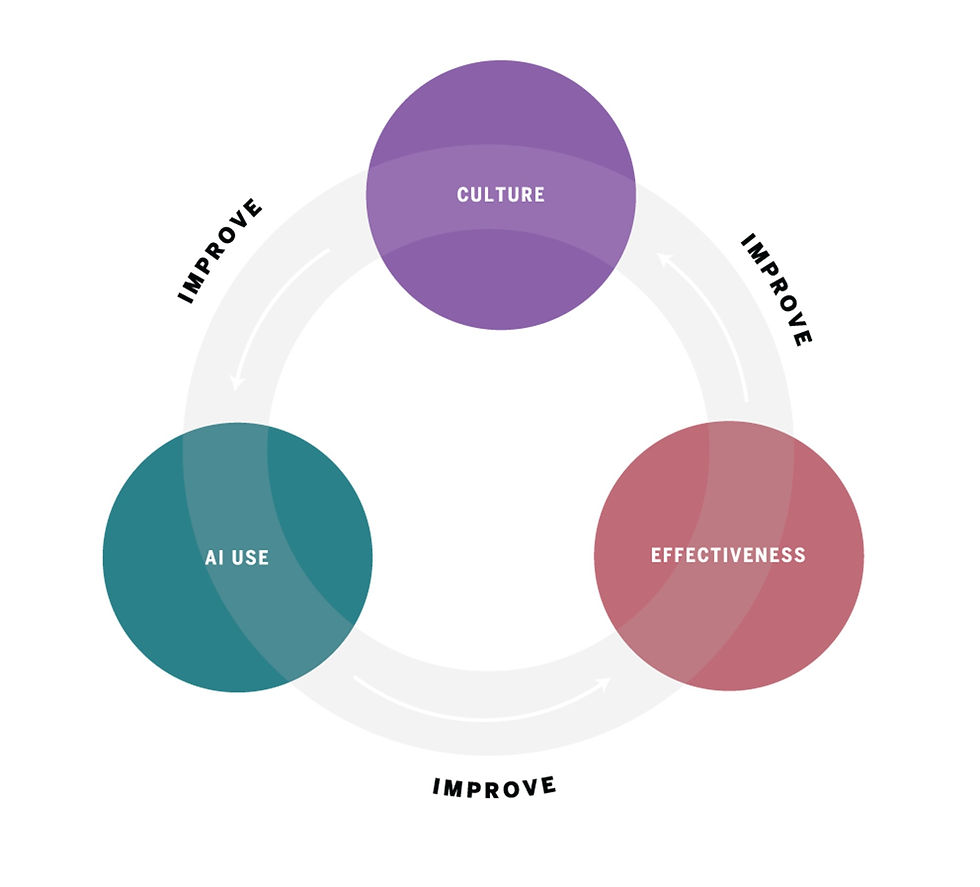
Image source: MIT Sloan Review
AI Usage is part of a feedback loop that transforms team culture and mentality.
This use case illustrates multiple benefits of AI-based methodologies. Namely, it shows the potential for AI to save a team’s time and better optimize decision-making. However, it also hints at something a bit more subtle that AI can deliver to organizations of all types: a change in mentality.
Once again, by empowering improved decision-making at every level of an organization, AI can create a more agile, collaborative environment. This environment is also less dependent on hierarchical decision-making processes that, while still necessary in other instances, can sometimes slow action, experimentation, and the discovery of solutions.
This is the power of AI. It doesn’t offer cookie-cutter solutions, because innovation isn’t a one-size-fits-all affair. Instead, it enables organizations to build connections between teams and develop bespoke solutions that solve issues unique to their own business processes.
Straight Talk on AI: What It Can’t Do (Yet)
Of course, for all that AI can do to revolutionize both what businesses can do and how they do it, there’s still plenty that it doesn’t offer. Along with assessing AI’s possibilities, understanding the limits of AI is important to set realistic goals and mitigate risk. Here’s a rundown of things that AI still can’t achieve.
AI Can’t Always Explain Its Decisions
Even though humans are responsible for developing the algorithms and neural networks that AI leverages for analysis, that doesn’t mean they always get to see exactly how they’re put to use.
This problem of “explainability” is nothing new in AI. However, it is an issue that’s growing: as our datasets and models become more complex, it becomes harder and harder for AI to explain in human terms how it arrived at a certain decision.
This conundrum has given rise to the field of explainable AI (XAI), which is focused on developing transparent AI models that can help address ethical and legal concerns surrounding how AI makes decisions. AI leaders like IBM and Google each offer toolkits to help organizations implement more transparent AI with confidence.
AI Can’t Be Creative (Independently)
AI that can replicate human intelligence is still something of a pipedream for researchers. That means a creative AI capable of making art and developing unique creative solutions all on its own is still something that will have to wait for the future.
As Dr. John Smith, an IBM Fellow at the IBM T. J. Watson Research Center describes it, “It’s easy for AI to come up with something novel just randomly. But it’s very hard to come up with something that is novel and unexpected and useful.”
So while AI has been used to successfully create movie trailers, songs, and even full-length novels, it still relies heavily on human-made parameters to do so.
AI Can’t Fully Replace Humans
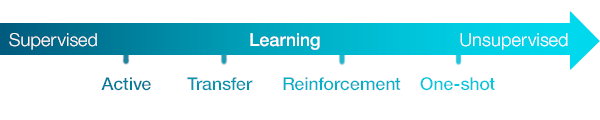
Image source: IBM
Along with creativity, the prospect of an AI that can learn without the supervision of humans is the ultimate goal of many researchers.
However, the possibility of moving beyond the mostly supervised machine and deep learning capabilities that we have now is still likely a long way off.
So, until then, the fact remains that AI always requires some degree of human input and guidance. This leaves businesses looking to drive innovation with two approaches to AI-augmentation and automation, which we’ll cover in-depth below.
AI Can’t Overcome Biases in Data and Algorithms
Just as AI can’t turn inadequate data into great insights, AI also can’t overcome biases (conscious or unconscious) that creep into that data, whether during data collection or selection.
The effects of this bias in AI can be devastating, particularly as trust in AI for decision-making grows. Negative outcomes of AI bias include everything from misinformed recruiting decisions and financial models, to errors with even more dire consequences like misrepresented medical prognoses and incorrect criminal-justice decisions.
Worse yet, because many are quick to trust AI-backed findings as “objective” or “data-driven,” the biases baked into data and analysis might more easily be overlooked.
The importance of taking responsibility for employing a more ethical AI can’t be overstated. As you explore the possibilities of artificial intelligence, combat biases along the way by:
Keeping up-to-date on research with resources from groups like the Partnership on AI and the Alan Turing Institute’s Fairness, Transparency, and Privacy group.
Establishing responsible processes that can mitigate bias. Google AI provides a series of recommended AI practices.
Diversifying the field of AI with investments in diverse talent, education, and advocates of advancement like AI4ALL.
AI Augmentation vs. Automation-And How to Activate Both

Image source: Towards Data Science
Thus far in our guides to AI and innovation, we’ve honed in on the basics of what AI can (and can’t) do for businesses. However, translating these examples into use cases for your own business still requires a deeper analysis of how AI gets things done.
Ultimately, AI capabilities boil down to two categories:
Automation: AI can replace human activity, freeing them up to focus on other tasks.
Augmentation: AI can support human activity and strengthen its outcomes.
As AI expert and developer Jacob Bergdahl writes, though, the vast majority of AI solutions actually incorporate aspects of both concepts. That is, automation vs. augmentation isn’t a cut-and-dry dichotomy: AI solutions exist on a spectrum somewhere between these two extremes. On this spectrum, Bergdahl writes, AI solutions can be further broken down into four key strategy types: efficiency, effectiveness, expertise, and innovation.
Understanding what separates these four strategies can help your business develop its own practical approach to AI development.
1. Efficiency Strategy
An efficiency strategy is one that leverages automation to optimize business activities. The goal of this optimization is often to cut costs. As we’ve discussed, automating repetitive and routine processes may also benefit businesses by freeing up talent to focus on more creative, collaborative activities.
Efficiency Strategy Use Cases
Contract Analysis: Deloitte developed the DocQMiner solution to help companies derive insights from unstructured documents. For instance, when a change in international regulations required that thousands of contracts be examined for compliance, DocQMiner was implemented, cutting analysis down to just minutes per document. Previously, it took an analyst about 90 minutes to review each contract.
Human Resources: Similarly, Deloitte has utilized its own smart robot, named Edgy, to optimize a wide range of HR processes. “Repetitive tasks performed according to fixed rules are easy to automate using RPA, or Robotic Processing Automation,” according to Henri Drogulski, Senior Consultant of HR Strategy & Service Delivery at Deloitte. In addition to analyzing CVs from job applicants and assisting with rote tasks like entering expense claims, the physical, humanoid robot is actually capable of interacting with Deloitte employees and potential candidates-welcoming people, answering questions, and even cracking the occasional joke.
Deloitte’s HR Robot, Edgy, in Action
2. Effectiveness Strategy
An effectiveness strategy draws on both automation and augmentation to improve the way workers communicate and coordinate with each other.
Effectiveness Strategy Use Cases
Effective Scheduling: The scheduling application described above is an example of an effective strategy in action. Similarly, consultancies like Deloitte utilize AI solutions to help determine to which consultant(s) projects should be assigned. The solutions can weigh parameters like skills, experience, and even employee preferences to quickly select the best candidate.
Chatbots: Chatbots are among the most common solutions developed according to an effectiveness strategy. Frequently, these solutions partially automate customer support, only passing customers on to live agents when a situation requires a human touch or a more complex solution. However, chatbots also make effective internal tools for a variety of businesses. Deloitte is helping its employees search its online technical library more efficiently by developing a smart chatbot.
Virtual Assistants: Meanwhile, common AI assistants like Siri and Alexa are built with the same strategy in mind-whether they’re being used by consumers in their homes or businesses in the field.
3. Expert Strategy
The expert strategy, at its heart, is about augmentation. Using this strategy, organizations deploy AI solutions with the goal of empowering and elevating human decision-making.
Expert Strategy Uses Cases
Financial and Risk Advisory: Financial advisors can leverage AI to help make better monetary decisions. Most of Wall Street now utilizes AI, whether to execute high-frequency trades more reliably or support investment decisions with advanced predictive analytics. Elsewhere, machine learning is helping advisors detect anomalous transactions and identify fraud.
Medical Imaging: Even medical professionals can use AI to aid their own expertise. For instance, doctors can find support while making diagnoses with AI-powered imaging technology.
4. Innovation Strategy
An innovation strategy, according to Bergdahl, is the most advanced of these AI strategies. Innovation relies on AI solutions to enable and enhance creativity.
Innovation Strategy Use Cases
Generative Design: An example of this strategy in action is generative design, which combines AI and cloud processing to help designers explore new engineering possibilities based on parameters developed by human-led teams.
Digital Writing Assistants: Meanwhile, AI is helping writers create more engaging (and grammatically correct) content. Popular applications like Grammarly spot typos and offer suggestions for clearer sentences on the fly. Even Gmail has incorporated Smart Compose, which uses machine learning to predict the next words a writer might include in their email. These same capabilities are helping creative writers generate video scripts, movie plots, and even full-length novels.
Improved Job Interview Experiences: Of course, creativity is critical to a wide variety of professional situations. Organizations like Modern Hire offer solutions, for example, that help job interviewers come up with creative follow-up questions in real-time.
Selecting the Right Innovation Strategy
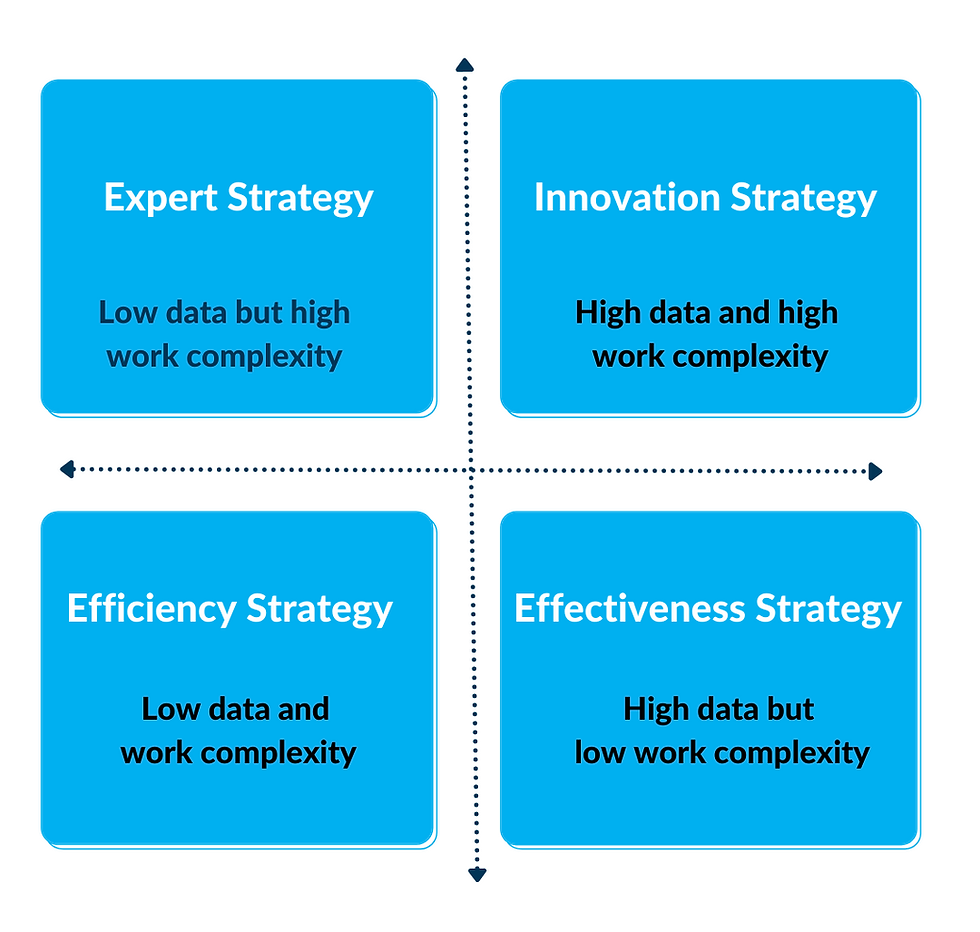
As the graphic above shows, these strategies don’t just exist on a spectrum between automation and augmentation. They’re also defined by their varying degrees of data and work complexity.
By understanding the relative complexity of the data and work involved in the activities your organization is analyzing, you can determine which AI strategy is right for your business. As Bergdahl describes:
Low data and work complexity = the efficiency strategy.
High data but low work complexity = the effectiveness strategy.
Low data but high work complexity = the expert strategy.
High data and work complexity = the innovation strategy.
Moving Toward Applied AI: The Practice of AI Strategic Planning
How, though, do you move from a general strategy to actual application of AI capabilities? This is a central question faced by many businesses today. The following study sheds light on a potential answer.
Manufacturing Industry Case Study Outlines Successful AI Planning Process
Surveying a wide range of manufacturing companies at different stages of their AI journey, a team of researchers developed a useful framework that can help guide AI strategic planning in an array of industries.
With their analysis, the team uncovered three interrelated capabilities whose development was essential for the manufacturers’ innovation success:
Data pipeline capabilities: In an industrial environment, data can be captured by numerous assets and sensors. To build sustainable AI solutions, then, developing a data pipeline through which data is collected, integrated, and securely managed provides a critical avenue for analysis and impact.
Algorithm development capabilities: These capabilities enable the creation of basic AI functions. Algorithms are developed to predict the “future state or actions of the business” based on incoming data from the firm’s pipeline. This development requires deep (typically external) expertise; each of the firms studied possessed partnerships with IT companies in order to access leading AI platforms.
AI democratization capabilities: Finally, these capabilities combine to focus on making AI accessible to the wider organization while demonstrating its potential. In short, to successfully innovate and transform with AI, the study affirms that organizations must translate initial capabilities into hunger for widespread adoption. This requires companies to actively identify additional valuable and concrete use cases while promoting a culture of experimentation and supporting personnel who attempt rapid trials with a “learn fast and fail fast” mentality.
Translating These Outcomes to All Industries
While tailored to the manufacturing industry, this study-which we’ll return to for even more insights in part 3 of our AI and Innovation series-has important lessons for businesses of all types. For a firm’s initial forays into AI, these insights sketch a framework with three key ingredients:
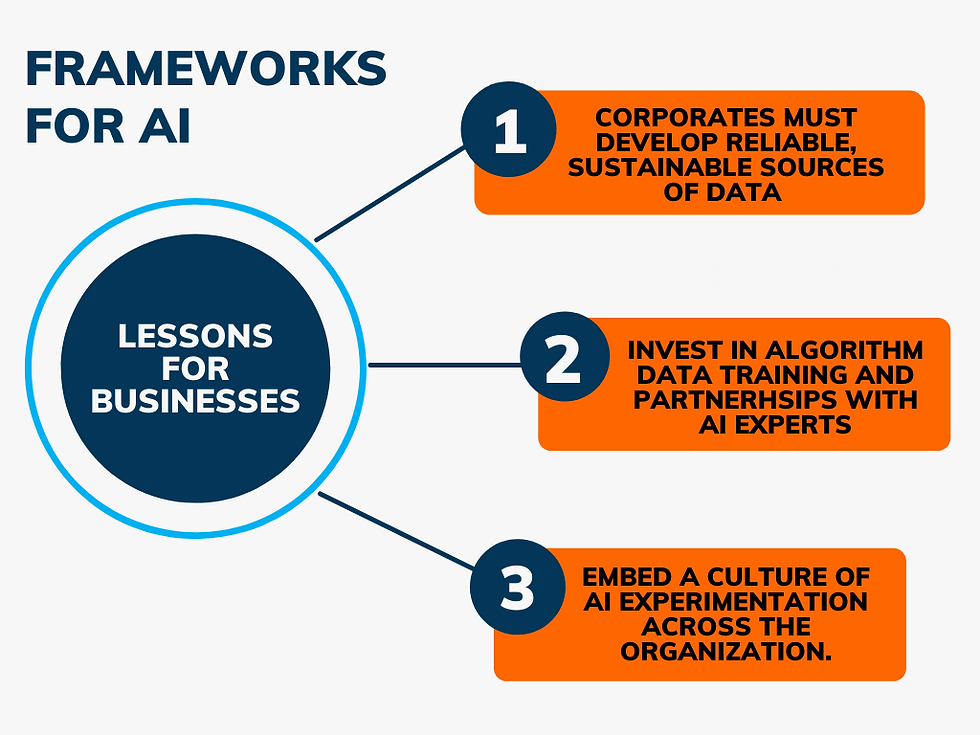
Corporates must develop reliable, sustainable sources of data.
They must invest in developing the algorithms that will be trained on that incoming data. Moreover, firms that do so successfully typically need to rely on external partnerships with AI experts.
Importantly, isolated use cases do not typically lead to widespread AI adoption and business transformation. That is, AI-backed innovation requires upfront investments in communication and evangelization to broadcast the value of AI, help others identify additional use cases, and embed a culture of AI experimentation across the organization.
Conclusion
In part one of our AI and Innovation series, we described the incredible potential of AI.
Now, with the help of the resources and insights described above, a practical path toward the innovative and sustainable use of artificial intelligence technologies and methodologies should come into much clearer view. By mapping your own organizational goals to the appropriate strategy, you can begin the process of envisioning concrete and valuable AI solutions.
Stay tuned, though. Because in part three of this series, we’ll go even deeper, getting into the nuts and bolts of practical AI application. By the end of the series, you’ll be ready to move from the key ingredients for AI solutions outlined above, to the process of creating your organization’s own comprehensive action plan for AI innovation.